Predictive Maintenance Protocols for Continuous Production Line Efficiency
Predictive Maintenance Fundamentals for Continuous Production
Core Components of Effective Protocols
Establishing a solid foundation for predictive maintenance demands identification of the core components essential for successful implementation. These typically include equipment condition monitoring, data analytics, and a maintenance strategy crafted to align with production goals. Condition monitoring involves tracking parameters such as vibration, temperature, and humidity to gain insights into equipment operation. By integrating condition-based maintenance practices, manufacturers can greatly enhance Overall Equipment Effectiveness (OEE) by minimizing unexpected failures and downtime. This proactive approach contrasts sharply with traditional reactive methods, converting historical data into actionable strategies, thus optimizing equipment performance.
IoT Integration for Real-Time Equipment Monitoring
IoT technologies are pivotal in enabling real-time equipment monitoring and providing vital data on machinery performance and health indicators. The use of sensors embedded in machinery facilitates continuous data collection, which can be crucial for intelligent decision-making in maintenance schedules. This approach is supported by industry insights suggesting that implementing IoT solutions can potentially reduce unplanned downtime by as much as 30%. Real-time data collection also empowers organizations to adopt proactive interventions, thus minimizing disruptions to production and enhancing operational continuity.
Machine Learning in Failure Prediction Systems
Machine learning algorithms offer significant advantages by analyzing historical data to discern patterns indicative of potential equipment failures. This capability shifts organizations from reactive to proactive maintenance strategies using predictive analytics to optimize resource allocation. According to research from leading tech experts, businesses leveraging machine learning for predictive maintenance can reduce maintenance costs by approximately 25%. By forecasting issues before they escalate, organizations can streamline maintenance processes and improve their overall efficiency and reliability in production environments. Integrating machine learning effectively not only prolongs the lifespan of machinery but also enhances productivity and safety.
Optimizing Footwear Manufacturing Efficiency
Reducing Unplanned Downtime in Leather Processing
Understanding and addressing the root causes of unplanned downtime in leather processing can significantly enhance production efficiency. Analyzing data from past incidents helps in developing targeted strategies that minimize such disruptions. Regular maintenance schedules, when aligned with predictive analytics, play a crucial role in minimizing unexpected interruptions. This proactive approach ensures machinery is in optimal condition, thereby reducing downtime. Evidence indicates that organizations focusing on maintenance activities can achieve up to 15% higher productivity, emphasizing the strategic importance of maintenance culture in industrial operations.
LSI Technologies: Precision in Shoe Stitching Workflows
Integrating advanced LSI technologies into shoe stitching workflows enhances precision and efficiency, leading to superior quality products. Modern sewing machines equipped with automated stitching controls are pivotal in achieving high accuracy, reducing human error, and expediting the manufacturing process. According to case studies, implementing precision technologies can result in a 20% reduction in material waste. Such savings not only cut costs but also contribute to sustainable manufacturing practices, making precision a key driver of both economic and environmental benefits in footwear production.
Case Study: Automotive Industry OEE Improvements
Lessons from the automotive industry's improvements in Overall Equipment Effectiveness (OEE) can greatly benefit the footwear sector. By embracing lean manufacturing principles and leveraging advanced analytics, automotive firms have witnessed a remarkable transformation in their production efficiency. Recent data reveals that effective OEE strategies have boosted production output by over 30%. This success illustrates the power of structured efficiency practices combined with data-driven decision-making, providing a blueprint for footwear manufacturers seeking to optimize their operational performance and maintain a competitive edge in the market.
TengHong Machinery’s Predictive Maintenance-Ready Solutions
TH-739B: 7-Pincer Computer Memory Control Toe Lasting Machine
The TH-739B model is engineered to enhance precision in toe lasting tasks through advanced computer memory control. This cutting-edge technology ensures exact repeatability, crucial for the demanding requirements of footwear manufacturing. The automated features of the TH-739B facilitate reduced cycle times, thus increasing operational throughput significantly. Sales data reveals that users of the TH-739B have experienced a 15% reduction in production costs, underscoring its efficiency in streamlining manufacturing processes.
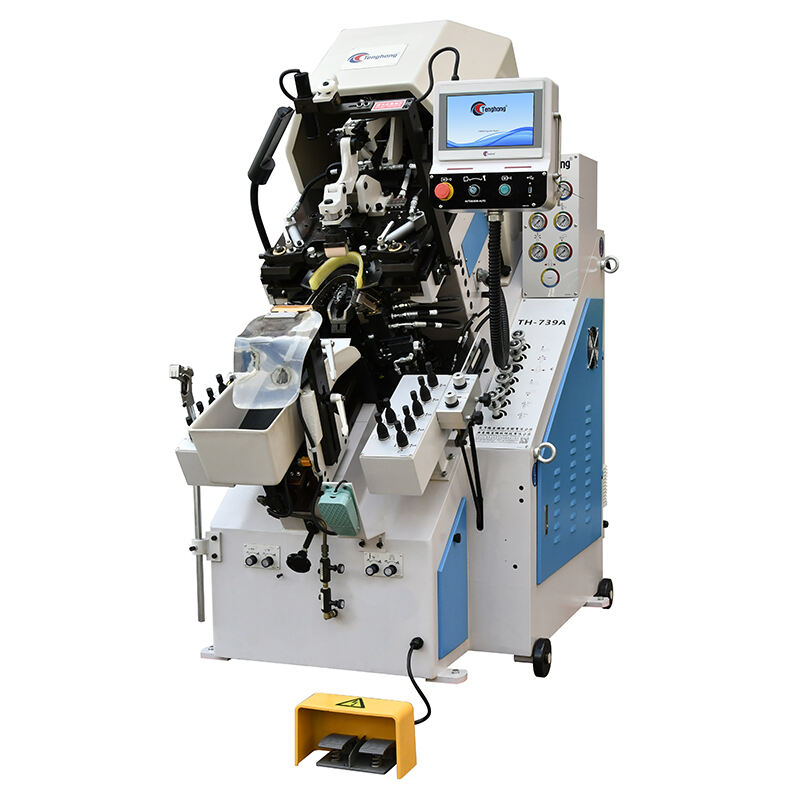
TH-747A: 9-Pincer Automated System with Error Diagnostics
The TH-747A is equipped with error diagnostic tools, making real-time troubleshooting during production seamless. This system allows operators to quickly spot and correct issues, maintaining high quality standards imperative for competitive manufacturing. User testimonials praise the TH-747A for considerably improving production efficiency, allowing manufacturers to uphold high standards without compromising speed.
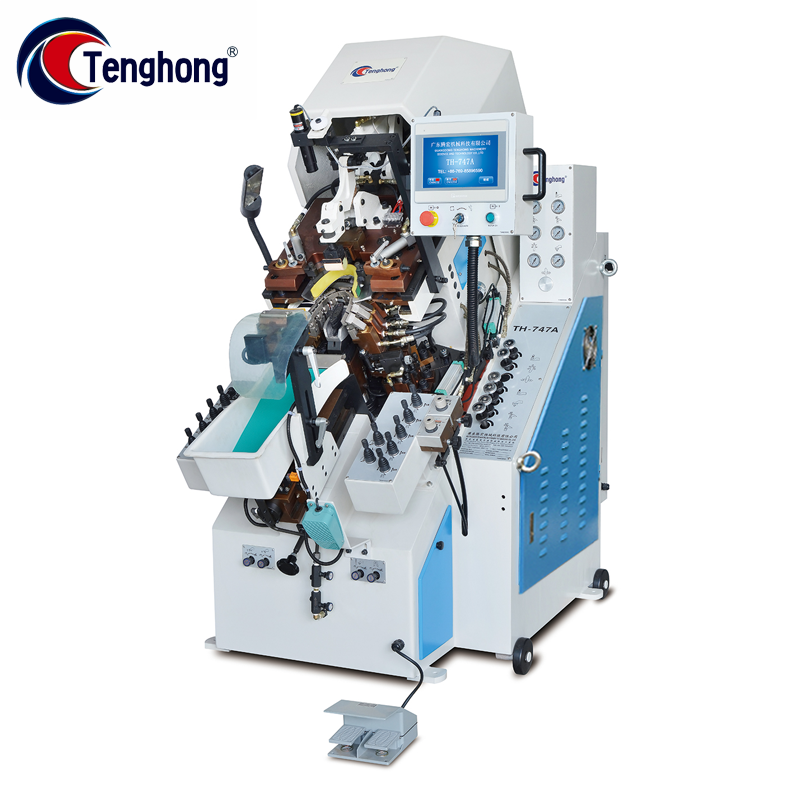
TH-747MA: Cementing Machine with Smart Process Controls
The TH-747MA holds smart process controls that enhance adhesive consistency, crucial in maintaining the integrity of footwear products. These intelligent controls streamline operations, reducing material waste and ensuring product quality remains uncompromised. Industry benchmarks show utilizing the smart machinery like TH-747MA can boost adhesive strength by 10%, enhancing both product durability and manufacturing efficiency.
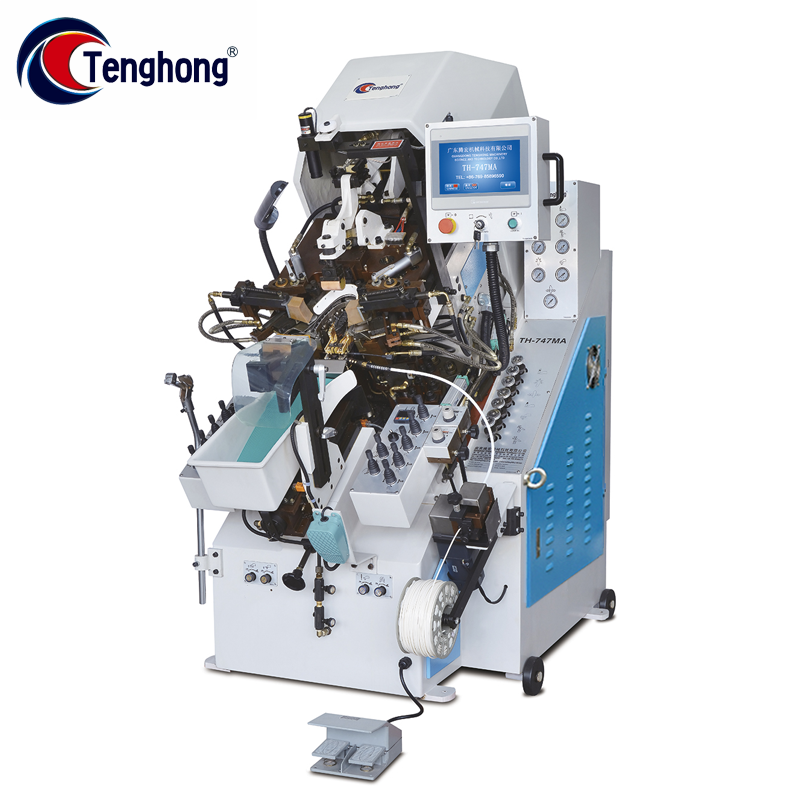
Implementing Protocols for Leather Processing Lines
Customizing Predictive Strategies for Clicker Press Operations
Customizing predictive maintenance strategies for clicker press operations can significantly enhance productivity in the leather processing industry. By identifying unique operational challenges, businesses can tailor these strategies to address specific production needs effectively. For instance, a predictive strategy could involve collecting data on a machine's wear and tear patterns, which helps in scheduling timely maintenance. Reports indicate that implementing customized maintenance plans has led to a 25% increase in machine uptime, demonstrating their potential to improve operational efficiency considerably.
Data-Driven Continuous Improvement Cycles
Establishing data-driven approaches fosters a culture of continuous improvement within the leather processing lines. Regular performance data analysis is crucial, as it helps pinpoint areas needing enhancement, thereby ensuring sustainable manufacturing practices. Longitudinal studies highlight the benefits of this approach, showing that organizations embracing continuous improvement see up to 20% gains in operational efficiency. By utilizing this strategy, leather processing lines can optimize their resource allocation while maintaining high production standards.
Integrating Leather Strip Cutter Analytics
Integrating analytics into leather strip cutter operations allows for optimizing cutting precision and material utilization. By capturing data during the cutting process, operators can make informed adjustments that enhance output quality and minimize waste. These analytics-driven improvement initiatives have been shown to yield approximately 15% less spoilage in output. By leveraging such technologies, leather processing lines can streamline operations, reduce costs, and achieve consistent product quality, contributing to overall manufacturing efficacy.
Overall, implementing these protocols across various stages of the leather processing line not only boosts efficiency but also promotes a sustainable and data-informed approach to manufacturing. By leveraging predictive strategies, data-driven improvements, and analytics, companies can ensure that their operations are both efficient and competitive.